Navigating the legal environment of AI (part 1)
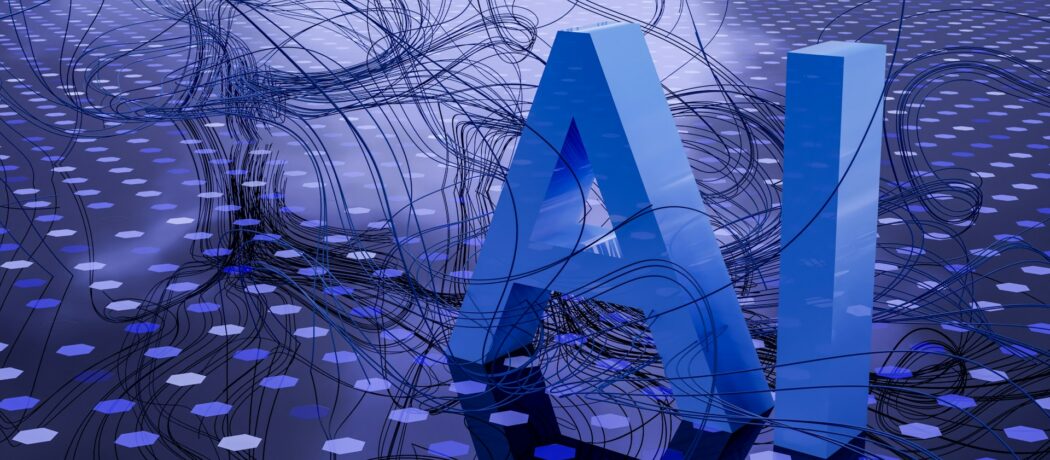
Share
As artificial intelligence (AI) continues to impact different business sectors and industries, companies are facing a complex landscape of legal challenges. The rapid adoption of this technology offers opportunities, but it also presents both legal and ethical risks that businesses cannot afford to overlook. In the first part of this interview with Maximiliano MARZETTI and Clare SHIN from IÉSEG we delve into the legal risks companies should anticipate and practical steps companies can take to ensure they comply with different regulations (relating to artificial intelligence).
What are the key legal risks companies should anticipate within the rapidly evolving AI legal landscape?
Maximiliano MARZETTI (MM): Understanding the legal environment of business is a key competence for any manager; not only to minimise legal risk, but also to leverage competitive advantages the legal system may provide. The AI market is not “unregulated” as some may think. Behind AI Systems (AIS) there are physical and legal persons (companies) who are responsible and subject to the law like everybody else.
However, whether existing general legal rules and theories (for instance, regarding torts or extracontractual liability) would suffice, or must be adapted to AI, remains to be seen. In any case, there are specific legal areas that AI companies should start paying particular attention to:
Data Protection and Privacy Laws: AI systems often require large amounts of data as inputs, which can include personal data. Ensuring compliance with applicable data protection laws, like the EU General Data Protection Regulation (GDPR) or the California Consumer Privacy Act (CCPA), is therefore crucial for companies.
Anti-Bias and Anti-Discrimination Legal Rules: AI systems can be biased and engage in illegal discriminatory practices, such as racial or gender discrimination. This does not need to be intended; it can be the consequence of limited training data or of the unconscious bias of a programmer. Whichever the case, to avoid liability and reputational harm, companies must take measures to minimise them, such as ensuring AI transparency and explainability.
Ad Hoc AI Laws: The recent AI boom, driven by technologies such as Large Language Models (LLMs) and Generative AI (GenAI), has heightened concerns about the potential intrinsic risks posed by advanced AIs. This has fuelled debates and led to the introduction of comprehensive, ad hoc AI laws, with the EU’s AI Act (AIA) being the epitome, aimed at addressing these challenges. However, despite some efforts, there is no uniform global approach to the regulation of AI systems. Anu Bradford (Columbia Law School) identifies three competing global regulatory models for regulating digital technologies, including AI: (a) the Market-based US Model, which adopts a laissez-faire perspective with minimal government intervention; (b) the State-directed Chinese Model, characterized by strict, centralized control over digital technologies and data; and (c) the Rights-based European Model, which emphasizes the protection of fundamental rights through strict regulations for tech companies; such as the AIA. This regulatory disparity may make compliance particularly difficult for companies operating in multiple jurisdictions, exposing them to conflicts due to contradictory legal rules and standards.
Intellectual Property and Trade Secret Law: Intellectual Property (IP) has been the area attracting the most litigation so far, particularly concerning copyright infringement claims related to the training of AI systems. The protection of algorithms through trade secrets, and the exceptions to such protection due to broader societal concerns, such as human rights violations or the enforcement of the rule of law, may also lead to future litigation.
Competition Law: The oligopolistic structure of the market for AI system development, dominated by a handful of firms, most of which are located in the US, may raise anticompetitive concerns. Some competition authorities are already closely monitoring this new market structure and issuing warnings or recommendations. For instance, the French competition watchdog recently issued an opinion on the GenAI market.
In this rapidly evolving and complex legal environment what practical steps can all companies (including those using artificial intelligence) take to ensure they comply with these regulations?
Clare SHIN (CS): Navigating the legal landscape of AI can be challenging, but there are concrete steps companies can take to establish compliance and mitigate risks. There are three different categories that companies should focus on: (1) Documentation, (2) Establishing an AI Governance team and policy, and (3) Training and awareness.
Thorough and precise documentation is foundational for companies aiming to comply with regulations such as the EU Artificial Intelligence Act (AIA) while maintaining a comprehensive understanding of AI utilization across all departments. Effective record-keeping practices enable organizations to respond swiftly in the event of a compliance issue or breach and provide a centralized repository for informed decision-making. Key elements of AI documentation should include the intended purpose of the AI system, the types of data involved, identified risks, and the measures taken to mitigate those risks.
The AIA specifically mandates several forms of documentation, such as technical documentation (Article 11), standard record-keeping (Articles 12 and 18), the EU Declaration of Conformity (Article 47), and registration with the EU AI Database (Article 49). These documents must be accurate, transparent, and subject to ongoing human oversight, with reviews conducted by the organization’s AI Governance Team to ensure alignment with regulatory requirements and internal policies.
Establishing an AI Governance Team is essential for creating a robust framework to oversee AI implementation and compliance. This team should consist of a diverse group of experts with multidisciplinary perspectives, enabling them to address ethical, legal, and operational challenges effectively. The team is responsible for drafting and enforcing clear policies on AI usage, developing strategies to ensure compliance with applicable regulations, conducting conformity assessments, and aligning practices with harmonized standards. They must also ensure transparency and ethical practices in AI applications.
For example, if an organization’s AI policy states, “We do not use AI to process sensitive client data,” the team must ensure strict adherence, even to the extent of prohibiting tools such as automated transcription services during online meetings involving sensitive discussions. These policies must be communicated clearly and enforced through comprehensive employee training programs.
In all cases, regardless of AI, companies must take the same basic steps to comply with regulations through training and monitoring, followed by remedial actions when any errors or deviations from the norm occur. AI must be developed, deployed, and corrected as if it were a human employee. Even with training, human employees can still make mistakes, and those mistakes must be identified and corrected.
Even though it is a machine, AI is similar to its human employee counterpart in that it can also deviate from the norms as it learns. Therefore, AI must be developed, deployed, and corrected as if it were a human employee. However, while the same general training, monitoring and corrective processes apply to humans and AI, the way in which they are carried out are different.
AI-specific training should begin during the development phase, with code designed to incorporate regulatory requirements and mitigate risks such as bias. Organizations adopting AI tools must ensure these systems align with their specific ethical and regulatory objectives. Like human employees, AI systems require initial qualifications, followed by ongoing modifications to meet evolving regulatory standards.
Equally important is training human employees to effectively and responsibly utilize AI. As tools like generative AI (e.g., ChatGPT) and AI-powered systems for decision-making become integral to daily operations, organizations must prioritize AI literacy. Training should include building awareness of privacy risks, such as avoiding the discussion of personal issues during AI-enabled online meetings, and understanding best practices for safeguarding sensitive company or client data, including refraining from directly inputting confidential information into generative AI systems.
By cultivating an AI-literate workforce and maintaining rigorous oversight of AI systems, organizations can navigate the complexities of the legal environment with confidence and integrity. This structured approach not only ensures compliance with regulatory frameworks like the AIA but also positions organizations to harness AI’s transformative potential responsibly and ethically.
AI systems often require large amounts of data. Can you explain how companies using AI (artificial intelligence) tools can seek to assure data protection and security?
CS: Indeed, AI is built on a foundation comprised of mass data. This means that data protection and security are paramount, not just as regulatory requirements but also as ethical imperatives and competitive advantages. When companies deploying AI tools handle vast amounts of sensitive data, including personal information, proprietary business data, and even national security-relevant information, it must ensure the protection and security of this data. Such responsibilities are critical for maintaining public trust, complying with legal obligations, and mitigating risks of reputational harm and financial penalties.
The importance of data protection in AI in essentially intertwined. Improperly managed data can lead to breaches, unauthorized access, and misuse, which can lead to actual human harm. This is why many jurisdictions have implemented regulations monitoring the safety of personal data within its borders, such as the EU with the General Data Protection Regulation (GDPR) and South Korea under the Personal Information Protection Act (PIPA). Non-compliance with these regulations can result in severe fines and operational restrictions. In addition to these personal data laws, these jurisdictions have also implemented regulations detailing specific requirements for high-risk AI systems, making data protection a core component of AI governance.
To assure data protection and security, companies using AI tools should adopt a comprehensive, flexible, and multidisciplinary approach that prioritizes the safety and rights of data subjects. For example, implementing data minimization and purpose limitation can help with the fundamental basis in data protection. Organizations should only collect and process the data that are strictly necessary for the intended purpose of the AI system. By doing so, they can reduce the risks associated with storing excessive data which can be compromised, while aligning with legal and ethical practices.
Equally important is the establishment of robust data governance frameworks. These frameworks provide clear policies and procedures for data handling, including data classification, access controls, and retention schedules, ensuring accountability and consistency across the organization.
Techniques such as anonymization and pseudonymization can further enhance data security by ensuring that even if personal data is accessed by unauthorized parties, it cannot easily be traced back to individuals. Regular risk assessments and impact analyses, such as Data Protection Impact Assessments (DPIAs), are also essential. These evaluations help identify potential risks and vulnerabilities in AI systems, enabling organizations to implement necessary mitigations proactively.
Companies can also embed security directly into its operation by utilizing concepts of privacy by design into the AI systems. This involves integrating security measures such as encryption, secure authentication protocols, and real-time monitoring for anomalies directly into the architecture of AI solutions. By addressing security from the beginning, companies can prevent vulnerabilities from becoming systemic issues later on.
Finally, when working with third-party vendors for AI solutions, companies must conduct thorough due diligence to ensure compliance with data protection standards. Contracts with vendors should include robust data protection clauses and allow for audits to verify compliance, and clear communication about how data is collected, processed, and protected should be shared with stakeholders to be transparent and build up trust and reputation.
The second part of this interview regarding artificial intelligence is available here:
Updates: Further developments up to 20 February 2025
By Maximiliano Marzetti
The first weeks of 2025 brought significant updates to the AI regulatory landscape. The law & geoeconomics of AI is in permanent change. Recent declarations and actions by the Trump administration in the US, since taking office, suggest that AI policy divergences across the Atlantic will continue to widen. On January 20, 2025, President Trump signed Executive Order No. 14148, revoking President Biden’s Executive Order of 2023, among others.
Just days later, the new President signed a new Executive Order (No. 14179) on Removing barriers to American Leadership in AI. While the US seems to be removing legal barriers to AI developments, the EU continues to implement its AI Act. However, the EU’s draft “code of practice” has faced significant criticism from major AI companies.
This divide was evident at the February Paris AI Summit, where over 60 countries and regional organizations, including China and the EU, signed the Statement on Inclusive and Sustainable Artificial Intelligence for People and the Planet. However, the US and the UK did not sign it. US Vice President Vance, who attended the summit, clearly stated the US stance, when he said that “we need an international regulatory regime that fosters the creation of AI technology, rather than strangles it.” Publishers and other copyright industries were not satisfied with the Paris Statement either. Even if it mentions “the need for a global reflection integrating inter alia questions of (…) intellectual property rights”, it does not, according to them, position copyright protection as a priority.
Additionally, the first decision on whether using copyrighted content for AI training qualifies as “fair use” has been issued. On February 11, judge Bias from the US District Court of Delaware (a first instance court), in the case between Thomson Reuters Enterprise Centre GmbH et al v. ROSS Intelligence Inc., unambiguously stated that the use of copyrighted works for AI training does not qualify as “fair use.”