Implementing interpretable Artificial Intelligence (AI) in an organization: an interview with professors Coussement and De Caigny
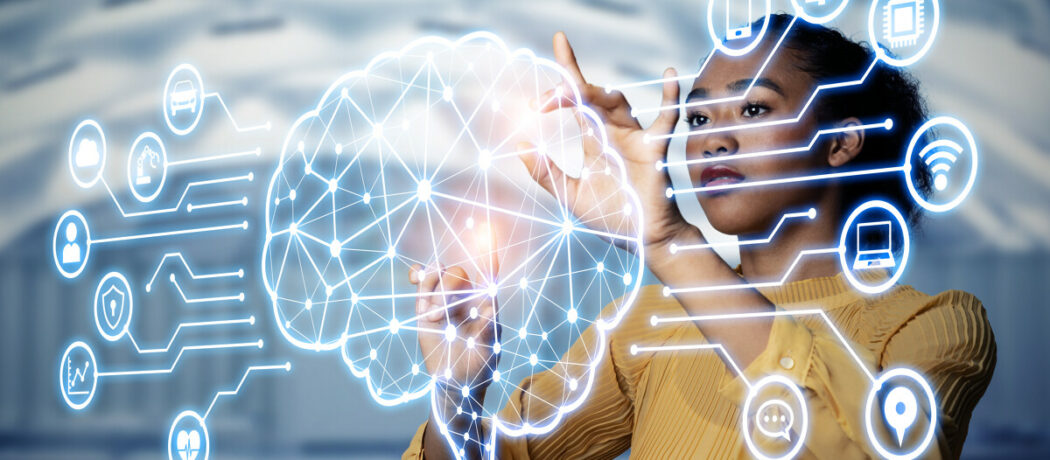
Share
Companies worldwide are increasingly turning to Artificial Intelligence (AI). Recent findings of a global survey by McKinsey & Company, for example, show that half of respondents reported that their organization had adopted AI in at least one business function (marketing, finance, etc.) and that some of these companies “plan to invest even more in AI in response to the COVID-19 pandemic and its acceleration of all things digital”.
Kristof COUSSEMENT, professor at IÉSEG School of Management and director of the IÉSEG center of excellence for marketing analytics (ICMA), and professor Arno DE CAIGNY are experts in big data analytics and the application of AI models in organizations. We spoke to them about their research into the development of interpretable AI (i.e. AI that can be used to provide and explain practical insights for improved business decision making) and asked them to give some concrete advice for organizations on its implementation.
Could you briefly explain what interpretable artificial Intelligence is and why it is important?
Kristof COUSSEMENT: AI and the use of data science continues to grow in importance. They are now deployed across a variety of business functions including marketing (for example with customer relation management and direct marketing) but also by finance for credit risk or fraud detection, as well as HR teams to improve recruitment or detect burn-out. There are several key components to consider to successfully implement AI: data in its different forms including numeric data and unstructured data such as text, audio, images for example; the various AI methodologies used to crunch this data (including machine learning and deep learning); and finally the insights and knowledge generated for organizations so value is added to their businesses. These are the three main pillars in the AI process.
We clearly see in our field that many organizations are nowadays focusing on the algorithmic side of AI trying to implement the most efficient and effective algorithms. We believe that this is certainly important but that companies should not just be looking at the algorithms themselves but should be focusing on understanding what they are doing and delivering effective insights and knowledge through these algorithms. This is where interpretable AI comes in to play as it refers to the possibility for business users to understand and explain the data science model in question and to capitalize on this knowledge for improved decision-making.
It therefore connects the technological and methodological side of data science with the business knowledge that exists within an organization. It is crucial that these go hand in hand to build trust within an organization. If you do not gain trust from people using your algorithms they will never be implemented effectively. Finally, this understanding of algorithms enables managers to act on this data and set up concrete plans of action and potentially avoid any biases that might be developed via an algorithm.
Can you explain the findings of some of your recent research?
Arno DE CAIGNY: We have published a number of papers in this area, but I would like to highlight one paper published at the end of 2018, which looks at interpretable AI in the field of customer relationship management *. This research focuses specifically on customer churn prediction, one of the key pillars of CRM. Put simply, churn refers to the rate a company loses customers for example when they stop buying products or services. Our paper is listed as one of the most cited publications since 2018 in the CNRS cat. 1 European Journal of Operation Research, a demonstration of the importance of this topic in today’s business and academic context.
We focused on the development of a new predictive tool that can be used to help managers reduce churn. There are two important components for such tools; the first one is predictive performance – identifying which customers are likely to leave. The second one is interpretability. This means that the model created should provide insights into the factors that might be driving customers away so that organization can put measures in place to improve customer retention.
Our model (the logit leaf model) combines both these elements; predictive performance and the interpretability of insights. It creates different segments of customers, which boosts the predictive performance and allows for interpretation of the drivers for churn on customer segment level rather than on the entire customer base.
Imagine for example you have two different types of customers that leave – those leaving because of price/cost factors and those who leave for example due to poor customer service. Hence, companies could benefit from targeting these different types of customers with different incentives to retain them. The logit leaf model allows organizations to achieve this goal by segmenting and separating groups of customers while traditional models have generally taken an overall approach for analyzing this problem.
What are the main messages that companies should bear in mind developing or implementing algorithms to enhance customer relation management?
Kristof COUSSEMENT and Arno DE CAIGNY: There are several messages that we would give to companies concerning interpretable AI.
1) The first relates to the richness and quality of the data. This is key and is the first step to successfully implementing interpretable AI in an organization. If you crunch poor data you will get very poor results and insights. It’s not just a question of quantity, it’s important to have a richness or diversity of data; not just numerical data but data potentially coming from call centers, social media, etc.
2) The second relates to the choice of algorithm. Data scientists often refer to black box and white box solutions. With the former, it is really a case of dropping in the data and the black box crunches the information without deeper knowledge on the process. While these are generally good for predictive performance, they do not provide the interpretable insights of a white box solution. It is important, therefore, that organizations carefully balance the choice of their algorithmic models. Of course, the model choice really depends on companies’ maturity in terms of data analytics. For example, if we consider startups or young companies that might not be working with data analytics, more interpretive models are likely to be more useful to convince the business users of its usefulness.
3) The presentation of the outcomes is crucial. Therefore, everything that deals with data visualization is key. The findings need to be easily understandable and explainable for managers in the organization.
4) The final point is that interpretable AI needs to be actionable. This means that the AI outcomes needs to provide insights to activities that can be put in place. For example, with customer loyalty schemes, models might provide clear insights into which groups of customers should potentially be moved into a more/less-rewarding program to drive engagement.
As a final consideration, interpretable AI requires having a “human” touch to the technological and methodological field of data science. For example over the last years various profiles in the field of data science were added to the traditional data scientist, data engineer or data analyst (roles). For instance, many organizations hire data strategists who are positioned between the business and the data science department. These data strategists have both the technical and methodological competences in AI and the in-depth understanding of a company’s strategy and vision.
ICMA
ICMA is the Center of Excellence for Marketing Analytics of IÉSEG School of Management. It is a knowledge hub formed by a team of academic experts with a proven track record in the fields of marketing analytics that aims to support teaching, research and business projects
*De Caigny, A., Coussement, K., & De Bock, K. W. (2018). A new hybrid classification algorithm for customer churn prediction based on logistic regression and decision trees. European Journal of Operational Research, 269(2), 760–772.